Increasing E&P Efficiency with the Cloud and Machine Learning
Paris, France | Jun 14, 2018
CGG GeoSoftware Introduces Cloud-Ready Applications and Python Plugins
The IT industry is experiencing an important transformation as companies invest in new technologies to drive growth and innovation. This trend is strongly reflected in our industry as E&P companies deal with enormous amounts of legacy, and increasing volumes of new data along with the expense and complexity of software to analyze and interpret this information. Challenges faced include operational efficiency, increasingly short project cycle times, communicating with a regional or global workforce, data silos, legacy software and restricted resources.
The Cloud
Due to the industry’s dynamics and its need for flexibility and information security, the cloud is increasingly seen as a viable and practical solution for the oil & gas industry, particularly now that Cloud service providers are building security into their software development processes. Security of data in the Cloud is often better today than in company’s own networks.
As part of this shift to the Cloud, GeoSoftware will be offering existing applications in the Cloud as well as continuing to make them available on currently supported platforms. In phase one, HampsonRussell and Jason will be available from a client’s Microsoft Azure Cloud. This includes compute-intensive GeoSoftware applications used for inversion and AVO analysis not only being multi-CPU enabled, but being able to access Cloud compute power. If a hundred-node cluster is needed to complete projects quickly, GeoSoftware applications can automatically provision this in the cloud, execute tasks in parallel and then tear down the virtual cluster to make sure that users only pay for the cloud infrastructure when needed. In addition, PowerLog can be accessed from the Cloud today through PetroCubic.
Future GeoSoftware developments will include commercialization with other Cloud providers like Amazon (AWS) and Google (GCP) and software re-design to take full advantage of the Cloud and provide a Software-as-a-Service model.
Machine Learning
PowerLog™ now provides access to machine learning capabilities that enable users to address complex petrophysical and reservoir engineering challenges. The native Python Extensions implementation in the latest version is a game-changer when it comes to developing workflows to address a wide variety of challenges including developing permeability models in carbonates, developing porosity and permeability models in unconventional reservoirs, generating missing log curves and data clustering for facies classification. PowerLog now enables users to take advantage of open-source Python utilities and programs which include hundreds of scientific calculation, data analysis and visualization libraries and programs.
Emerge from HampsonRussell also provides machine learning capabilities, an important tool for geoscientists, which can improve the accuracy of lithological predictions in seismic analysis. Developed more than 20 years ago, Emerge’s original neural network capabilities were well suited for geoscience challenges, given that they can solve for many variables at one time and handle the very complex statistical analysis required to create a prediction of lithology from large seismic data volumes. Today, Emerge has many types of supervised neural networks that focus on different kinds of problems. Supervised networks are first trained by presenting them with observed values (such as well porosity) at well tie locations and then letting them determine a relationship between the seismic attributes and the well values. An example of such a neural network would be the Kohonen self-organizing map. A chief distinction from deterministic seismic analysis methods such as inversion and AVO is that multivariate statistics, classification and neural networks do not assume an underlying model, but instead try to build the relationships directly from the data. This distinction is significant because it removes potential bias from the analysis.
Attendees can visit CGG booth #1640 to hear about recent developments in Cloud computing and Machine Learning from GeoSoftware experts and how new approaches can bring added value to existing work practices, processes, and workflows. Live demos of PowerLog Machine Learning for Facies Classification and daily booth theater presentations cover these topics.
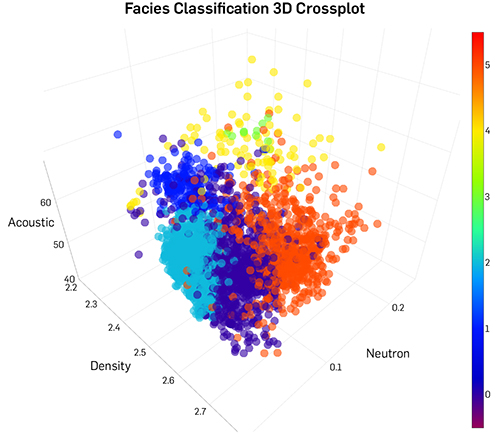
About CGG
CGG (www.cgg.com) is a global geoscience technology leader. Employing around 3,700 people worldwide, CGG provides a comprehensive range of data, products, services and solutions that support our clients to more efficiently and responsibly solve complex natural resource, environmental and infrastructure challenges. CGG is listed on the Euronext Paris SA (ISIN: 0013181864).